Our world, with all its modern conveniences, is built on batteries. Batteries have arguably been the bedrock for significant technological advancements that revolutionized the way we live. Innovations in batteries enabled passenger vehicles, mobile phones and laptops, and even the international space station. This will be increasingly true going forward, with consumer devices getting more powerful, automakers focusing heavily on electrification, and renewable energy generation levels increasing quickly.
The global demand for batteries is projected to exceed 1800 GWh by 2030, driven largely by the push towards electric vehicles (EVs). However, the lingering question for many experts in the field is:
Can the cost of battery capacity decrease enough to really enable mass-market electric vehicles and grid storage?
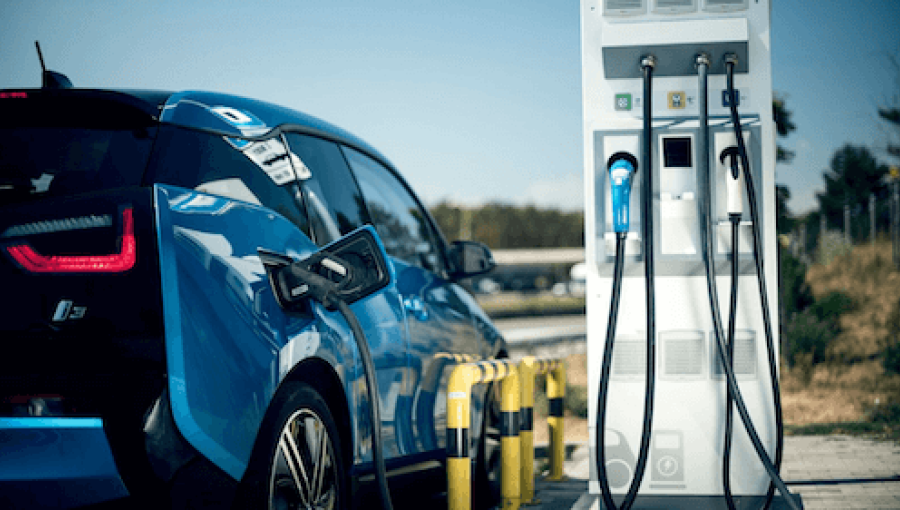
Innovation has focused much more on new materials and chemistries to improve energy and power density. While energy density is an important factor for cutting cost per kWh, efficiency and consistency in battery production processes is an important (and often overlooked) part of the equation. To date, battery production has been more of an art than a science. In order to meet the projected global demand at a reasonable cost, the industry must also focus on improving production processes to ensure quality and avoid wasting time and resources.
Currently, a significant amount of scrap is generated during manufacturing, as many process errors and defects go undetected until the battery production process is almost complete. If we can detect these issues earlier, we can help turn more input material into usable product and lower the overall cost of production. Additionally, detecting defects and errors earlier reduces the risk of battery failures in the field. We’ve all seen manufacturer recalls of cell phones, laptops, and electric vehicles to battery risks. These recalls ultimately end up indirectly driving up the cost of production for subsequent product batches.
By no means are manufacturers currently being careless and purposely letting cell defects go undetected. The issue boils down to one simple fact: the inside of a battery cell is still a black box. With today’s measurement methods, it’s very difficult to determine a battery cell’s state with certainty. Simply put, the time is now to create more accurate ways to inspect processes to ensure high levels of consistency and quality.
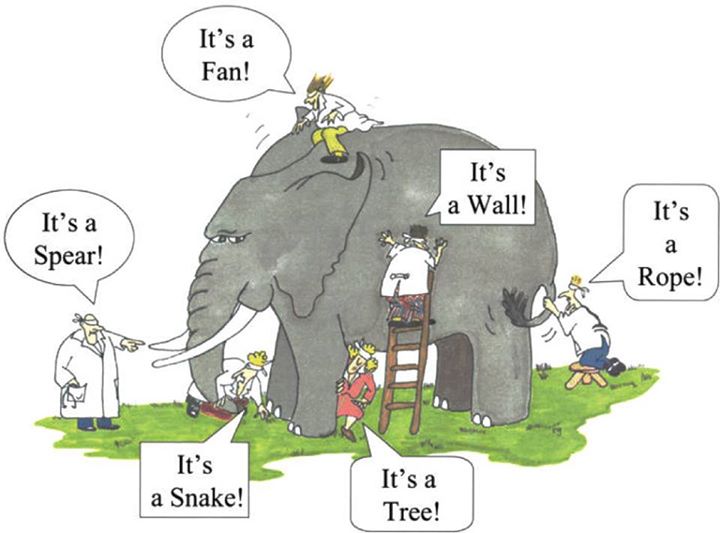
Is there way to see into batteries and detect errors, defects, or risks well before they become a problem?
Our team at Feasible believes we’ve found the answer. Using the powerful combination of ultrasound and machine learning we can gain insights like never before.
Imagine you are at the doctor’s office and they measure your blood pressure and pulse. These are critical vital signs but are surface deep and not sufficient for diagnosing serious illnesses before it is too late — you would certainly hope to be screened on a deeper level so you can take preventative measures. It’s no wonder that the advent of medical imaging and advanced clinical chemistry led to significant increases in life expectancy — doctors finally had the tools to take early action.
For batteries, electrical voltage and current are the main ways to determine physical condition at commercial scales. Just like blood pressure and pulse for humans, these are surface deep methods. More specifically, electrical methods give signals that are an average of the electrochemical reactions that occur across the entire area of a battery’s electrodes. Why is this a problem?
Consider the diagram below, which shows a defect (red dot) in a small and a large battery cell. In the smaller cell (e.g. for a phone or smartwatch), the defect occupies just meaningful fraction of the cell’s area, so its presence will be more easily detected with electrical signals. With larger cells (e.g. for an EV), however, the problem is exacerbated as the defect occupies a much smaller fraction of the entire cell’s area. These types of ambiguities are the root cause of many manufacturing inefficiencies and unexpected battery performance issues (and sometimes catastrophic failures in the field). With battery cells trending towards larger form factors and more demanding performance requirements, it is increasingly important to obtain deeper and more precise insights at all stages of cell and pack production.
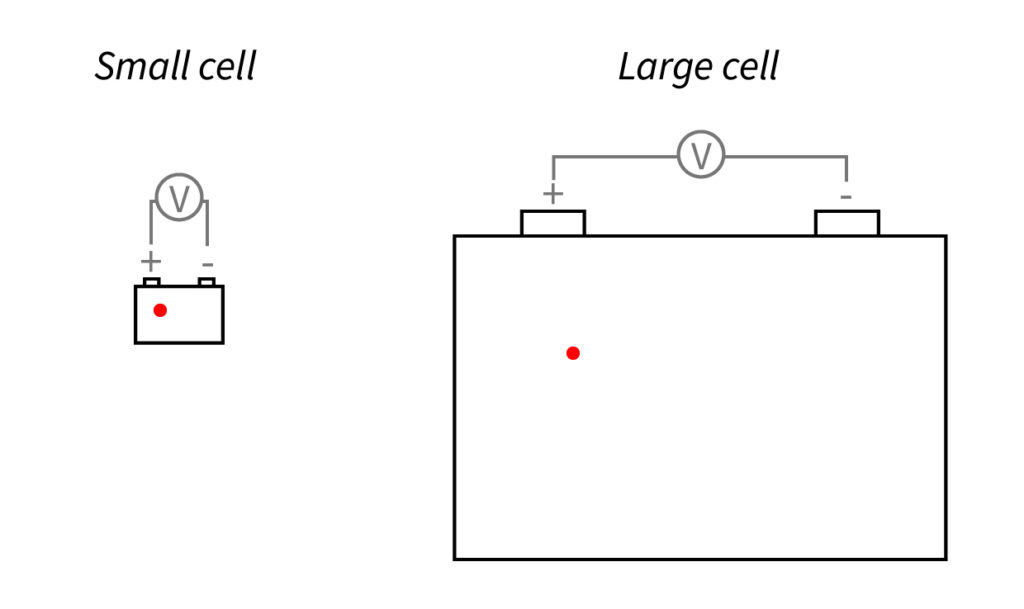
Analogous to medical imaging for the body, our EchoStat platform uses ultrasound and machine learning to deliver deeper physical insights about batteries that go well beyond the capabilities of conventional of voltage and current measurements. Ultrasound allows us to directly measure the physical structure of batteries and is sensitive to even the most subtle changes in the properties of internal components. EchoStat can quickly visualize the distribution of these properties throughout every cell, helping us understand everything that goes on inside a battery at every point along the value chain. It even allows us to predict the performance of the cells over time with great accuracy.
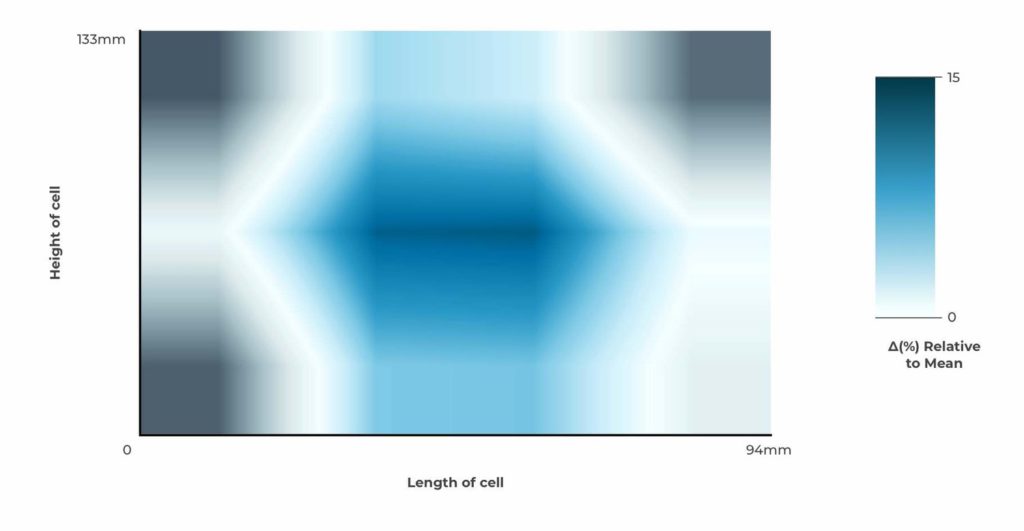
At Feasible, we believe that advanced inspection during battery production is critical for the industry to keep up with global demand for affordable EVs and higher levels of renewable energy generation. Our goal is to enable safer, more reliable, and better-performing batteries that are produced at lower costs. In doing so, we are paving the path for new technologies and are accelerating the transition to a clean energy future.
Feasible Overview: Advanced Battery Intelligence using Ultrasound and Machine Learning
What’s in a name?
Many people ask about the origin of our name. Fun fact — it’s an acronym that we used in an early grant funding proposal: Fast Electrochemical Acoustic Signal Investigation for Battery Lifetime Extension